Unlocking Potential: The Importance of Data Labeling in Machine Learning
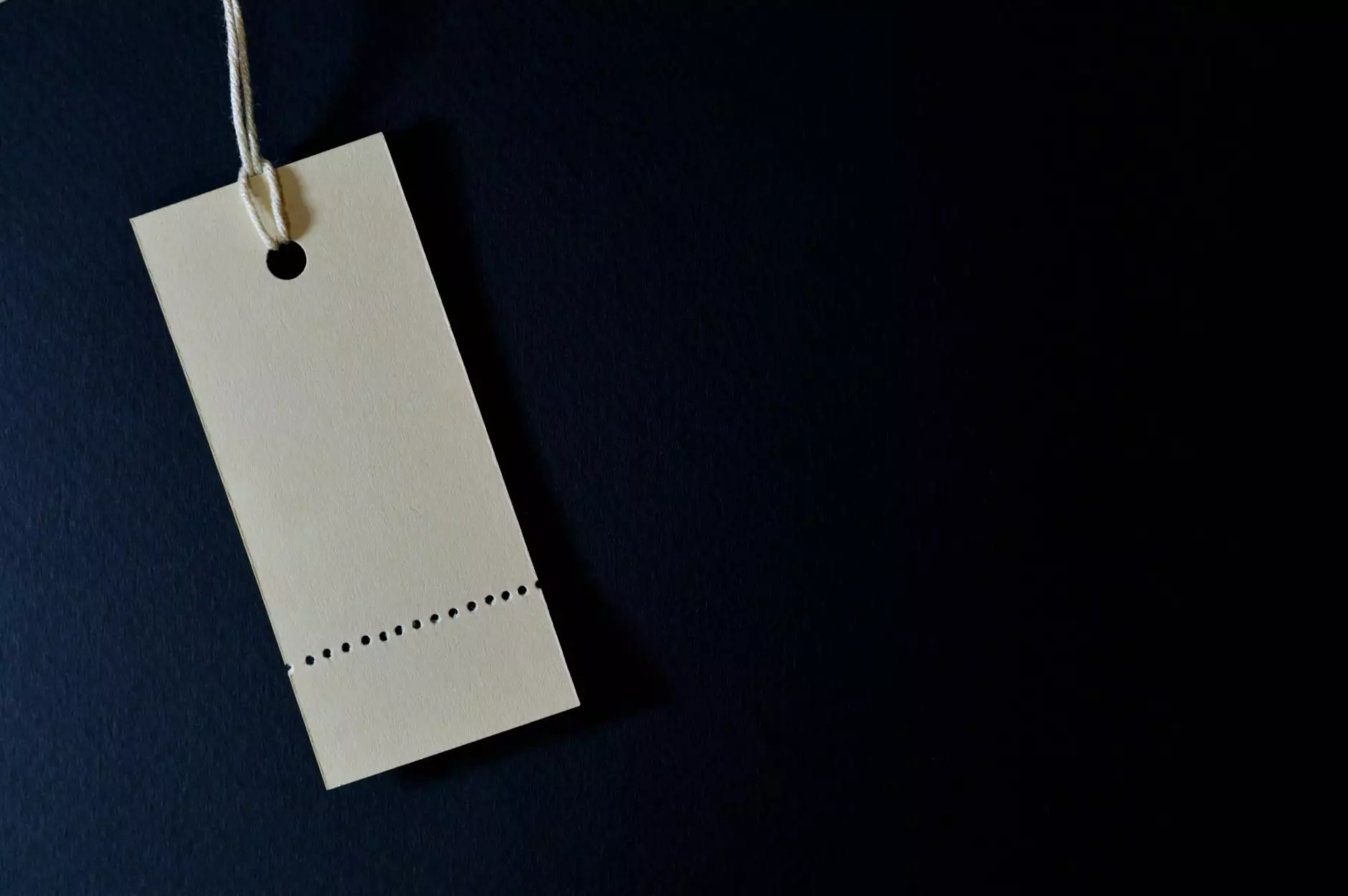
In today's rapidly evolving technological landscape, machine learning stands out as a powerful tool shaping multiple sectors. However, the effectiveness of machine learning algorithms hinges significantly on one crucial aspect: data labeling. This article seeks to delve into the nuanced world of data labeling machine learning and explore its relevance in diverse industries, especially for businesses like Keymakr, that thrive on precision services such as locksmithing and home security solutions.
Understanding Data Labeling in Machine Learning
At its core, data labeling is the process of identifying raw data and adding informative tags to it, which allows machine learning models to understand and interpret the data effectively. Whether it’s images, text, or audio, comprehensive labeling helps the algorithms learn from patterns and make predictions. Here are the fundamental types of data labeling:
- Image labeling: This involves annotating images with specific tags, bounding boxes, or pixel-level classifications. For instance, in the locksmith industry, image labeling can help automate the recognition of specific lock types.
- Text labeling: Categorizing text data into classes enables sentiment analysis, topic detection, and entity recognition. Businesses can leverage this to analyze customer feedback.
- Audio labeling: Identifying key features or components in audio data, which can be crucial for voice recognition systems used in personal security devices.
The Role of Data Labeling in Machine Learning Applications
Data labeling is pivotal across various sectors, including finance, healthcare, and particularly in home services like locksmithing. Here’s how effective data labeling drives growth and efficiency:
1. Enhancing Accuracy of Machine Learning Models
Quality labeled data directly correlates with the accuracy of machine learning models. A well-labeled dataset ensures that the model can differentiate between various inputs. For a locksmith service like Keymakr, having precise images of different locks labeled accordingly can enhance their inventory management systems and improve customer service.
2. Streamlining Operations
Automated systems that utilize machine learning can significantly streamline operations. For example, data labeling can help in developing smart automation tools for locksmiths that predict demand trends or identify common service requests based on historical data.
3. Improving Customer Experience
With advanced machine learning algorithms processing well-labeled data, businesses can provide tailored customer experiences. For Keymakr, utilizing machine learning to analyze customer interactions can lead to more effective communication and service offerings based on specific customer needs.
Data Labeling Processes: Best Practices
Implementing an effective data labeling machine learning strategy involves understanding best practices that ensure high-quality annotations:
1. Define Clear Guidelines
Creating a comprehensive set of guidelines for data annotators is essential. For example, in labeling images of locks, the guidelines should instruct annotators on what features to highlight, such as the keyhole, brand, and lock type.
2. Use Skilled Annotators
Utilizing professionals who understand the domain is crucial—this allows for a level of expertise that can mitigate errors during the labeling process. In the locksmith field, trained professionals can provide insights that a casual annotator might miss.
3. Employ Quality Assurance Measures
Establishing a robust quality assurance protocol, including spot checks and audits, ensures the accuracy of labeled data. Regular feedback loops can improve the quality and consistency of annotations over time.
The Future of Data Labeling in Machine Learning
As machine learning technologies continue to evolve, the methods of data labeling are also advancing. Here are some expected trends in the field:
1. Automation of Data Labeling
With the growth of AI, automating elements of the labeling process can save time and reduce human error. Tools powered by machine learning can assist in pre-labeling datasets that human annotators can refine.
2. Crowdsourcing Data Labeling
Leveraging crowdsourcing platforms for data labeling allows businesses to accelerate their processes while ensuring diverse input. It also reduces costs significantly, making it easier for smaller firms to access high-quality labeled data.
3. Real-Time Data Labeling
Future innovations may allow for real-time data labeling, where data is annotated as it is collected. This would be particularly beneficial in fields such as security and locksmith services, where rapid responses are vital.
Conclusion: Embracing Data Labeling for Business Growth
The importance of data labeling machine learning cannot be overstated, particularly for businesses like Keymakr that thrive on accuracy and efficiency in home services. By adopting comprehensive data labeling strategies, businesses can not only enhance their operational capabilities but also uplift the overall customer experience. As the machine learning landscape continues to grow, staying ahead of the curve with quality labeled data will unlock new potentials for innovation and success in the marketplace.
Call to Action
If you're looking to elevate your business operations through data labeling and machine learning, consider partnering with experts in the field. Whether you are in home services or another industry, the right data strategies can transform your operations and lead to exceptional growth.